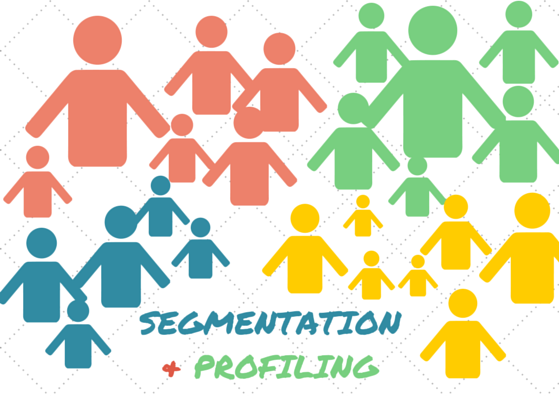
Have you ever wondered how your customers are behaving on a grand scale? Do you know which customers are most valuable to you and which customers represent an undiscovered opportunity? You might have a general idea about the behaviour of your customers - having observed their behaviour in the past - but are you sure your observations are supported by the facts or the data?
Last week my colleague Bram Vanschoenwinkel gave an extensive overview of the analytical techniques available for marketing and their added value. This week, I'll provide further detail on segmentation and profiling.
Customer segmentation and profiling is a technique that allows us to use customers’ behaviour - together with other more or less static characteristics such as demographics - as a means to categorise them into similar groups. The idea is that even though your customers and their actions might look completely random and scattered, patterns can be discerned among them.
Imagine walking through a supermarket with or without a grocery list. The things that you are going to buy might look or feel random to you in the breadth and width of the product assortments. To the supermarket however, your purchases can somewhat be anticipated by your previous behaviour and the behaviour of customers who are and behave ‘like you’.
Why should I care?
Knowing who your customer is and how he or she behaves is crucial to any business success. Competitive advantage comes from capitalising on uniqueness. Only when you know your customers' intricacies and behaviour, can you act upon their wishes while keeping up with emerging trends. A good example is Kodak. Kodak has filed for bankruptcy in 2012 after years of negative financial returns. Unfortunately, they were unable to see or acknowledge their customers' enthusiasm for newly emerging digital camera technology and wrongly assumed that their loyal customer base would stay.
Procter and Gamble is an example of a company that does know their target audience very well and highlights this throughout its communications. P&G produces a variety of products for the household, so naturally one of their target personas is the person in charge of buying these items. This quite often turns out to be the mom and the following commercial illustrates how they hit the heart strings of everyone watching, while communicating the value they bring to their target audience.
[embed]https://www.youtube.com/watch?v=5KlS45U7-O4[/embed]
How do I start understanding my customer?
In a classic setting, segmentation of a customer base usually happens with limited input from the business or the people that work with your customers on a daily basis. The data scientist would collect your data, do his magic and present you with a segmentation that might be plausible but doesn’t seem completely compatible with your gut feeling.
A more business focused way of segmenting your customer base can be achieved by first visualising the relationships between your customers in terms of their behaviour and constantly feeding back to the business what was discovered during the segmentation process. The ultimate goal and last step of the segmentation is then to profile these segments so that we get a clear description and mental picture of the type of customers presented by the different segments.
A recent case we have worked on illustrates the potential of this method. The project was conducted for a company that organises professional trainings and courses for other companies or professionals across a wide range of subjects. The goal of our analysis was to create a profile of ‘good’ customers. Good was described in terms of frequency and monetary value, i.e. how often does the customer attend a course and how much do they spend on training.
1. Visual Cluster Analysis
Starting with a visual analysis of the variables and plotting variables on a heat map using a technique called self organising maps, we can quickly validate whether the data confirms or denies the ‘hunches’ of the business.
The different nodes on each heat map contain the same customers, nodes closer to each other indicate similarity between the customers in these nodes. The further apart the nodes are, the more dissimilar the customers contained within the nodes. Red nodes for a particular variable indicate a high concentration of these types of customers in that node. White nodes indicate that there are no customers in that node with that characteristic.
In the above example, we can conclude that good customers are companies with a medium number of employees and are mostly not interested in HR courses. This information is then visually presented to the business to get confirmation on or to educate the business about previously unknown patterns in the data.
2. Interactive Segmentation
The next step is to use this information to cluster customers in similar groups based on the variables identified earlier. This is done using a clustering algorithm that maximises the similarity within one cluster and minimises the similarity between different clusters based on the variables describing the population.
The term interactive is used because it is paramount that this happens together with the business, to find out for instance, what number of clusters makes sense from a business perspective .
3. Profiling
Profiling is the third and last step and sets this method apart from traditional segmentation processes. The aim of this step is to create a profile or a description for each cluster identified earlier, focusing on the specific target set out in the beginning of the project. We use variables that were used in the clustering algorithm and descriptive variables not used for the actual clustering.
The output of this step is a clear description of each cluster, relative to each of the other clusters. We then focus on the cluster that contains most of the 'target' customers.
For this particular project, we were mostly interested in finding and describing good customers, which in this case is cluster 5. We called this cluster the 'Top of the crop'.
We confirmed with the business that the members of this cluster were indeed their customers perceived as 'best'. By plotting the number of customers in each clusters against the total amount spent per cluster we were able to see how valuable the 'Top of the crop' cluster actually was for the business.
So what?
We then continued to further analyse the 'Top of the crop' cluster and found that even though a big part of good customers was contained within 'Top of the crop', there were some customers who were not-yet a good customer according to the definition of the business. But as a result from the analysis, these almost-good customers pose a great potential. They are the ideal target for a marketing campaign as the segmentation has identified them as similar to good customers, they might just need a little push to become 'good' according to the business definition.
To conclude, this case study illustrates that you don't need to collect a lot of data to know your customer, nor do you need to invest heavily in specialist data analysis software. The data already available within your company is usually enough to make a head-start in understanding your customer and identify groups with similar characteristics. We implemented this three-step innovative segmentation method entirely in the open source tool R (The R Project for Statistical Computing), using a number of readily available libraries like kohonen and NbClust. These libraries can be freely downloaded from the Comprehensive R Archive Network (CRAN). Credit also has to go out to my former professor and now good friend, prof. Dr. Bart Baesens, whose research team published the paper ''A new SOM-based method for profile generation: Theory and an application in direct marketing'' from which we drew inspiration for the development of this method.
Like with any analytics project, rapid prototyping and daring to start small is key to create a competitive advantage. Nothing is stopping you to start analysing the data available to you today and create some simple but achievable models or analyses. Reaching a minimum lovable product quickly sets you and your company up for creative thinking and collaboration. Also, first results might be just what you need to convince stakeholders and managers of the potential opportunities hiding in your data.
“A good plan violently executed now is better than a perfect plan executed at some indefinite time in the future.”
George S. Patton Jr.
Other points to remember:
- Apart from rapid prototyping, it is also important to have an ambitious end-game. You can work towards it in increments: think big, act small.
- Involve the business early on and make sure they are engaged throughout the entire process. The business is your customer and ultimately has to believe and understand your model or analysis. Make sure you understand their pains and problems exactly. Do not make assumptions.
- Good data is the key to success and useful data is better than big data. Make sure the data you have is clean, relevant and up to date.
- Do not be afraid to experiment with different methods. Try different techniques for different purposes, do not just follow text book examples.
- Data, models, gut feeling and intuition are the perfect mix, do not leave out any of these ingredients.
Ready up!
Interested in learning more about this and other marketing analytics topics? Want to get practical experience? We regularly provide training sessions, in-company coaching and a lot more.
Knowing your customer and acting upon their needs and wants can go a long way in adding direction to your strategy and might fuel further analyses and research.
Because at the end of the day, your business depends on happy customers...