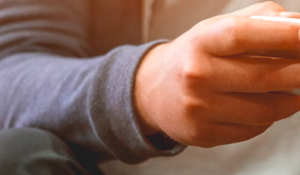
Artificial Intelligence (AI) and Machine Learning (ML) used in the right way offer companies new and unprecedented opportunities to tap into. For the man and woman in the street it brings many new and exciting advances in their everyday lives. However, they also have a dark side. When we design algorithms and train models based on data of a privileged minority group, ML model outcomes (like predictions, recommendations, classifications,...) have the ability to discriminate and exclude large parts of the population in a way that is not acceptable. Fact is that we don’t always realize this, and thus need to be very careful and build in checks to avoid these circumstances.
Bias in Machine Learning
Machine Learning bias is a phenomenon that occurs when an algorithm produces results that are systemically prejudiced due to erroneous assumptions in the ML process. That ML process often consists of the development of algorithms, and using these algorithms to train models from data. For example, the algorithms that are used by Netflix to train models to recommend you movies and series based on your historical usage data, i.e. the movies/series you watched before.
We make a distinction between two types of bias:
-
Algorithm bias. This occurs when there's a problem within the design of the algorithm that performs the calculations that power the ML computations and model training.
-
Data bias. This happens when there's a problem with the data used to train the ML model. In this type of bias, the data used is not representative enough to teach the system.
Let's consider the Netflix example for an illustration of data bias. One could decide not to include the time of the day in the historical data that is used to train the recommendation model. This would lead to a recommendation model that misses out on the fact that the user typically prefers different content at different times of the day. This is called exclusion bias, because some information is excluded from the data that is used to train the model.
Another common type of bias is sample bias. Sample bias occurs when a dataset does not reflect the realities of the environment in which a model will run. Let's consider the Netflix example once more to illustrate the idea. Typically Netflix also offers you recommendations based, not only on your own usage history, but also that of others. The recommendation "If you like this series, you will probably also like these series." typically stems from a model that learned these preferences from the historical usage data of a collection of users. In a ML context we call this collection of users, the sample on which a model will be trained. If this sample is chosen carelessly, this can lead to undesired results. For example, the sample may contain too many users of a certain nationality or language area. This results in the model learning a preference for movies and series in a certain language or from a certain country. Or, if the sample contains much more men than women, the model may prefer content for a typically male audience.
Discriminating AI
Machines do not have an intrinsic ability to let their personal experiences, opinions or beliefs influence their decisions. Machine Learning bias generally stems from problems introduced by the individuals who design and train the ML systems. Such biases can result in degraded model performance, like lower customer service experiences or reduced sales and revenue. Next to degraded performance another painful side effect is that it can also lead to unfair and potentially discriminating outcomes like discriminating women when recommending Netflix content as discussed in the example above. Such gender bias is unfortunately an all too common problem in ML systems, as are racial bias and various other forms of social bias. Let's have a look at some infamous examples.
Gender bias. In 2018, Reuters broke the news that Amazon had been working on a secret AI recruiting tool that showed bias against women. Here is an extract of the Reuters article:
Amazon.com Inc’s AMZN.O machine-learning specialists uncovered a big problem: their new recruiting engine did not like women. […] The team had been building computer programs since 2014 to review job applicants’ resumes with the aim of mechanizing the search for top talent […] In effect, Amazon’s system taught itself that male candidates were preferable. It penalized resumes that included the word “women’s,” as in “women’s chess club captain.” And it downgraded graduates of two all-women’s colleges, according to people familiar with the matter. They did not specify the names of the schools.
Amazon’s models were trained to evaluate applicants by observing patterns in resumes submitted to the company over a 10 year period. Most came from men, a reflection of male dominance across the tech industry.
Racial Bias. Last year there was a lot of commotion after a new tool for creating realistic, high-resolution images of people from pixelated photos showed its racial bias, turning a pixelated yet recognizable photo of former President Barack Obama into a high-resolution photo of a white man. Soon more images popped up of other famous Black, Asian, and Indian people, and other people of colour, being turned white.
Social bias. In 2020 a George Washington University study of 100 million Chicago rideshare trips concluded that a social bias in dynamic pricing existed depending on whether the pick-up point or destination neighborhood contained higher percentages of non-white residents, low-income residents or high-education residents.
Part of the problem is that there are relatively few black people working in AI. At some of the top technology companies, the numbers are especially grim: black workers represent only 2.5% of Google’s entire workforce and 4% of Facebook’s and Microsoft’s. Gender comparisons are also stark. A few weeks ago my colleague Valerie Taerwe wrote an interesting blog on female representation in the IT sector: the actual numbers show that the ratio of women is at 17% in Belgium. For the domain of AI a 2018 World Economic Forum study estimates that globally only 22% of AI professionals are female, while 78% are male.
Toward inclusive AI
These biases are often unintentional, but nevertheless the consequences of their presence in ML systems can be significant. So where do they come from? And more importantly, what can we do about it?
Our brain automatically tells us that we are safe with people who look, think and act similar to us. We are hard-wired to prefer people who look similar, sound similar and have similar interests. This is called unconscious bias. These preferences bypass our normal, rational and logical thinking. It was our survival trick on the savannah and still is useful most of the time today, but in many other situations it can lead to undesirable behaviour not in line with the norms and values of our contemporary worldview.
To prevent such undesired behaviours, there is a simple guideline of five points that companies can consider to overcome discrimination and achieve more inclusion in AI and ML:
-
Be conscious about the unconscious bias. Awareness is the first step towards a solution to the problem. If we are aware of the unconscious bias, we can anticipate it and prevent its unwanted effects.
-
Embrace diversity in workforce and leadership. If an organization’s leadership and workforce do not reflect the diverse range of customers it serves, its outputs will eventually be found to be substandard. Given that AI solution development depends on human decisions and by design, human beings have several unconscious biases, bias can creep in anywhere in the pipeline. If the diversity within an organization’s pipeline is low at any point, the organization opens itself up to biases.
-
Model evaluation should include an evaluation by social groups. No matter your team representation, you can work on inclusion by making your control group diverse. This is a generally applicable principle for everything companies do. Specifically within AI and ML it means we should strive to ensure that the metrics used to evaluate ML models are consistent when comparing different social groups, whether that be gender, ethnicity, age, social class, etc.
-
The data that one uses needs to represent “what should be” and not “what is”. Randomly sampled data will have biases because we live in a world that is inherently biased where equality is still a fantasy. Therefore we have to proactively ensure that the data we use represents everyone equally and in a way that does not cause discrimination against a particular group of people.
-
Accountability, responsibility and governance are key. As both individuals and companies have a social responsibility, they have an obligation to regulate ML and AI processes to ensure that they are ethical in their practices. This can mean several things, like installing an internal compliance team to mandate some sort of audit for every ML algorithm/model created. (A practice that is already widely used with privacy committees in the context of privacy, for example.)
Organisations that take into account the above guideline when designing, implementing and applying AI and ML can take an important step towards a more inclusive AI in which no minority (or majority) groups are excluded or disadvantaged in any way.